Personalized Depression Treatment
For a lot of people suffering from depression, traditional therapies and medication are ineffective. A customized treatment may be the answer.
Cue is an intervention platform that transforms sensor data collected from smartphones into customized micro-interventions for improving mental health. We analyzed the most effective-fit personal ML models for each subject using Shapley values to discover their feature predictors and uncover distinct features that deterministically change mood with time.
Predictors of Mood
Depression is a major cause of mental illness around the world.1 Yet only half of those affected receive treatment. To improve outcomes, healthcare professionals must be able to identify and treat patients who have the highest chance of responding to specific treatments.
The ability to tailor depression treatments is one method to achieve this. Researchers at the University of Illinois Chicago are developing new methods to predict which patients will benefit the most from certain treatments. They use mobile phone sensors as well as a voice assistant that incorporates artificial intelligence, and other digital tools. Two grants worth more than $10 million will be used to discover the biological and behavioral indicators of response.
So far, the majority of research on predictors for depression treatment effectiveness has focused on the sociodemographic and clinical aspects. These include demographic factors such as age, gender and education, clinical characteristics including symptoms severity and comorbidities and biological indicators such as neuroimaging and genetic variation.
Few studies have used longitudinal data in order to predict mood of individuals. Few studies also consider the fact that mood can vary significantly between individuals. It is therefore important to develop methods which allow for the determination and quantification of the individual differences in mood predictors treatments, mood predictors, etc.
The team's new approach uses daily, in-person evaluations of mood and lifestyle variables using a smartphone app called AWARE, a cognitive evaluation with the BiAffect app and electroencephalography -- an imaging technique that monitors brain activity. The team will then create algorithms to identify patterns of behaviour and emotions that are unique drugs to treat depression and anxiety each individual.
The team also created an algorithm for machine learning to identify dynamic predictors of each person's deep depression treatment mood. The algorithm combines these individual variations into a distinct "digital phenotype" for each participant.
This digital phenotype was correlated with CAT DI scores, a psychometrically validated severity scale for symptom severity. The correlation was low, however (Pearson r = 0,08; P-value adjusted by BH 3.55 x 10 03) and varied significantly among individuals.
Predictors of symptoms
Depression is among the leading causes of disability1, but it is often underdiagnosed and undertreated2. Depression disorders are rarely treated due to the stigma that surrounds them and the lack of effective interventions.
To facilitate personalized treatment to improve treatment, identifying the patterns that can predict symptoms is essential. Current prediction methods rely heavily on clinical interviews, which aren't reliable and only reveal a few features associated with depression.
Using machine learning to integrate continuous digital behavioral phenotypes captured through smartphone sensors and a validated online mental health tracker (the Computerized Adaptive Testing Depression Inventory the CAT-DI) together with other predictors of severity of symptoms has the potential to increase the accuracy of diagnostics and treatment efficacy for depression treatment free. These digital phenotypes allow continuous, high-resolution measurements as well as capture a wide range of unique behaviors and activity patterns that are difficult to document through interviews.
The study included University of California Los Angeles (UCLA) students with mild to severe depressive symptoms participating in the Screening and Treatment for Anxiety and Depression (STAND) program29, which was developed under the UCLA Depression Grand Challenge. Participants were referred to online support or clinical care according to the severity of their depression. Participants who scored a high on the CAT-DI scale of 35 or 65 were assigned to online support via the help of a peer coach. those with a score of 75 were routed to clinics in-person for psychotherapy.
Participants were asked a series of questions at the beginning of the study concerning their psychosocial and demographic characteristics as well as their socioeconomic status. The questions included education, age, sex and gender and financial status, marital status and whether they were divorced or not, their current suicidal thoughts, intent or attempts, and the frequency with which they consumed alcohol. Participants also scored their level of depression symptom severity on a scale of 0-100 using the CAT-DI. The CAT-DI tests were conducted every week for those who received online support and every week for those who received in-person treatment.
Predictors of Treatment Response
Research is focusing on personalization of depression therapy Treatment for depression. Many studies are focused on identifying predictors, which will aid clinicians in identifying the most effective medications to treat each patient. Particularly, pharmacogenetics can identify genetic variants that influence how the body's metabolism reacts to antidepressants. This lets doctors select the medication that will likely work best for each patient, while minimizing the amount of time and effort required for trial-and error treatments and avoiding any side consequences.
Another option is to build predictive models that incorporate clinical data and neural imaging data. These models can then be used to determine the best combination of variables predictive of a particular outcome, such as whether or not a particular medication is likely to improve the mood and symptoms. These models can be used to predict the response of a patient to treatment, allowing doctors maximize the effectiveness.
A new generation uses machine learning techniques such as supervised and classification algorithms such as regularized logistic regression, and tree-based methods to combine the effects of multiple variables and improve predictive accuracy. These models have been demonstrated to be useful in predicting the outcome of treatment, such as response to antidepressants. These methods are becoming popular in psychiatry and it is expected that they will become the norm for future clinical practice.
Research into the underlying causes of depression continues, as do predictive models based on ML. Recent findings suggest that the disorder is associated with dysfunctions in specific neural circuits. This theory suggests that individual depression non medical treatment for depression will be based on targeted therapies that target these circuits to restore normal function.
One method of doing this is by using internet-based programs which can offer an individualized and tailored experience for patients. One study found that a web-based program improved symptoms and improved quality of life for MDD patients. A randomized controlled study of a personalized treatment for depression revealed that a significant number of patients saw improvement over time and had fewer adverse effects.
Predictors of adverse effects
In the treatment of depression, a major challenge is predicting and identifying which antidepressant medication will have no or minimal negative side effects. Many patients are prescribed various medications before finding a medication that is effective and tolerated. Pharmacogenetics offers a fascinating new way to take an effective and precise approach to choosing antidepressant medications.
A variety of predictors are available to determine the best antidepressant to prescribe, including gene variants, patient phenotypes (e.g. gender, sex or ethnicity) and comorbidities. However it is difficult to determine the most reliable and valid predictive factors for a specific treatment will probably require randomized controlled trials with significantly larger numbers of participants than those that are typically part of clinical trials. This is because it could be more difficult to identify the effects of moderators or interactions in trials that contain only one episode per participant rather than multiple episodes over a period of time.
Furthermore, the estimation of a patient's response to a particular medication will also likely need to incorporate information regarding symptoms and comorbidities in addition to the patient's previous experience with tolerability and efficacy. Currently, only a few easily assessable sociodemographic variables and clinical variables appear to be consistently associated with response to MDD. These include gender, age, race/ethnicity, SES, BMI and the presence of alexithymia.
Many issues remain to be resolved in the use of pharmacogenetics in the treatment of depression. First, a clear understanding of the genetic mechanisms is required as well as an understanding of what is a reliable predictor of treatment response. In addition, ethical concerns like privacy and the ethical use of personal genetic information, must be carefully considered. In the long term, pharmacogenetics may provide an opportunity to reduce the stigma that surrounds mental health care and improve treatment outcomes for those struggling with depression. As with all psychiatric approaches it is essential to carefully consider and implement the plan. For now, the best course of action is to offer patients various effective depression medications and encourage them to speak with their physicians about their experiences and concerns.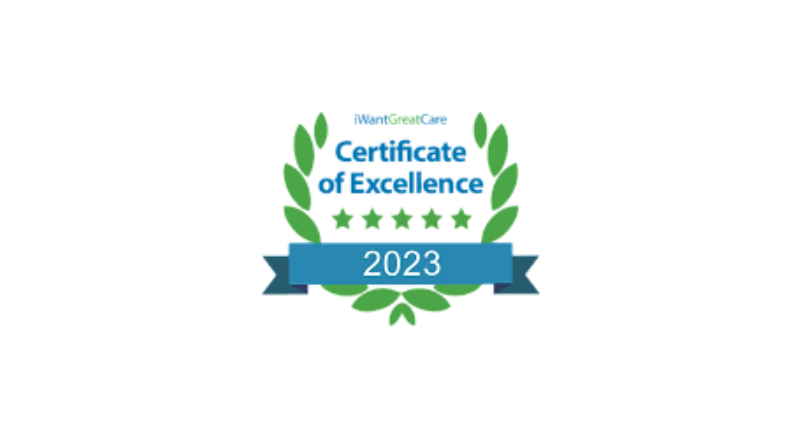
For a lot of people suffering from depression, traditional therapies and medication are ineffective. A customized treatment may be the answer.
Cue is an intervention platform that transforms sensor data collected from smartphones into customized micro-interventions for improving mental health. We analyzed the most effective-fit personal ML models for each subject using Shapley values to discover their feature predictors and uncover distinct features that deterministically change mood with time.
Predictors of Mood
Depression is a major cause of mental illness around the world.1 Yet only half of those affected receive treatment. To improve outcomes, healthcare professionals must be able to identify and treat patients who have the highest chance of responding to specific treatments.
The ability to tailor depression treatments is one method to achieve this. Researchers at the University of Illinois Chicago are developing new methods to predict which patients will benefit the most from certain treatments. They use mobile phone sensors as well as a voice assistant that incorporates artificial intelligence, and other digital tools. Two grants worth more than $10 million will be used to discover the biological and behavioral indicators of response.
So far, the majority of research on predictors for depression treatment effectiveness has focused on the sociodemographic and clinical aspects. These include demographic factors such as age, gender and education, clinical characteristics including symptoms severity and comorbidities and biological indicators such as neuroimaging and genetic variation.
Few studies have used longitudinal data in order to predict mood of individuals. Few studies also consider the fact that mood can vary significantly between individuals. It is therefore important to develop methods which allow for the determination and quantification of the individual differences in mood predictors treatments, mood predictors, etc.
The team's new approach uses daily, in-person evaluations of mood and lifestyle variables using a smartphone app called AWARE, a cognitive evaluation with the BiAffect app and electroencephalography -- an imaging technique that monitors brain activity. The team will then create algorithms to identify patterns of behaviour and emotions that are unique drugs to treat depression and anxiety each individual.
The team also created an algorithm for machine learning to identify dynamic predictors of each person's deep depression treatment mood. The algorithm combines these individual variations into a distinct "digital phenotype" for each participant.
This digital phenotype was correlated with CAT DI scores, a psychometrically validated severity scale for symptom severity. The correlation was low, however (Pearson r = 0,08; P-value adjusted by BH 3.55 x 10 03) and varied significantly among individuals.
Predictors of symptoms
Depression is among the leading causes of disability1, but it is often underdiagnosed and undertreated2. Depression disorders are rarely treated due to the stigma that surrounds them and the lack of effective interventions.
To facilitate personalized treatment to improve treatment, identifying the patterns that can predict symptoms is essential. Current prediction methods rely heavily on clinical interviews, which aren't reliable and only reveal a few features associated with depression.
Using machine learning to integrate continuous digital behavioral phenotypes captured through smartphone sensors and a validated online mental health tracker (the Computerized Adaptive Testing Depression Inventory the CAT-DI) together with other predictors of severity of symptoms has the potential to increase the accuracy of diagnostics and treatment efficacy for depression treatment free. These digital phenotypes allow continuous, high-resolution measurements as well as capture a wide range of unique behaviors and activity patterns that are difficult to document through interviews.
The study included University of California Los Angeles (UCLA) students with mild to severe depressive symptoms participating in the Screening and Treatment for Anxiety and Depression (STAND) program29, which was developed under the UCLA Depression Grand Challenge. Participants were referred to online support or clinical care according to the severity of their depression. Participants who scored a high on the CAT-DI scale of 35 or 65 were assigned to online support via the help of a peer coach. those with a score of 75 were routed to clinics in-person for psychotherapy.
Participants were asked a series of questions at the beginning of the study concerning their psychosocial and demographic characteristics as well as their socioeconomic status. The questions included education, age, sex and gender and financial status, marital status and whether they were divorced or not, their current suicidal thoughts, intent or attempts, and the frequency with which they consumed alcohol. Participants also scored their level of depression symptom severity on a scale of 0-100 using the CAT-DI. The CAT-DI tests were conducted every week for those who received online support and every week for those who received in-person treatment.
Predictors of Treatment Response
Research is focusing on personalization of depression therapy Treatment for depression. Many studies are focused on identifying predictors, which will aid clinicians in identifying the most effective medications to treat each patient. Particularly, pharmacogenetics can identify genetic variants that influence how the body's metabolism reacts to antidepressants. This lets doctors select the medication that will likely work best for each patient, while minimizing the amount of time and effort required for trial-and error treatments and avoiding any side consequences.
Another option is to build predictive models that incorporate clinical data and neural imaging data. These models can then be used to determine the best combination of variables predictive of a particular outcome, such as whether or not a particular medication is likely to improve the mood and symptoms. These models can be used to predict the response of a patient to treatment, allowing doctors maximize the effectiveness.
A new generation uses machine learning techniques such as supervised and classification algorithms such as regularized logistic regression, and tree-based methods to combine the effects of multiple variables and improve predictive accuracy. These models have been demonstrated to be useful in predicting the outcome of treatment, such as response to antidepressants. These methods are becoming popular in psychiatry and it is expected that they will become the norm for future clinical practice.
Research into the underlying causes of depression continues, as do predictive models based on ML. Recent findings suggest that the disorder is associated with dysfunctions in specific neural circuits. This theory suggests that individual depression non medical treatment for depression will be based on targeted therapies that target these circuits to restore normal function.
One method of doing this is by using internet-based programs which can offer an individualized and tailored experience for patients. One study found that a web-based program improved symptoms and improved quality of life for MDD patients. A randomized controlled study of a personalized treatment for depression revealed that a significant number of patients saw improvement over time and had fewer adverse effects.
Predictors of adverse effects
In the treatment of depression, a major challenge is predicting and identifying which antidepressant medication will have no or minimal negative side effects. Many patients are prescribed various medications before finding a medication that is effective and tolerated. Pharmacogenetics offers a fascinating new way to take an effective and precise approach to choosing antidepressant medications.
A variety of predictors are available to determine the best antidepressant to prescribe, including gene variants, patient phenotypes (e.g. gender, sex or ethnicity) and comorbidities. However it is difficult to determine the most reliable and valid predictive factors for a specific treatment will probably require randomized controlled trials with significantly larger numbers of participants than those that are typically part of clinical trials. This is because it could be more difficult to identify the effects of moderators or interactions in trials that contain only one episode per participant rather than multiple episodes over a period of time.
Furthermore, the estimation of a patient's response to a particular medication will also likely need to incorporate information regarding symptoms and comorbidities in addition to the patient's previous experience with tolerability and efficacy. Currently, only a few easily assessable sociodemographic variables and clinical variables appear to be consistently associated with response to MDD. These include gender, age, race/ethnicity, SES, BMI and the presence of alexithymia.
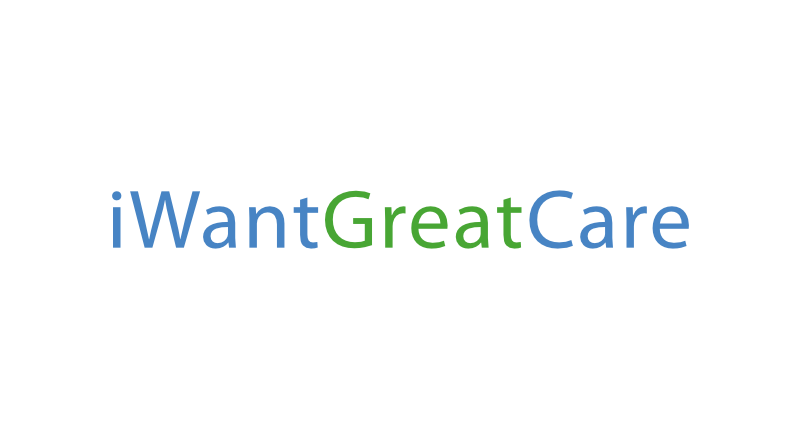
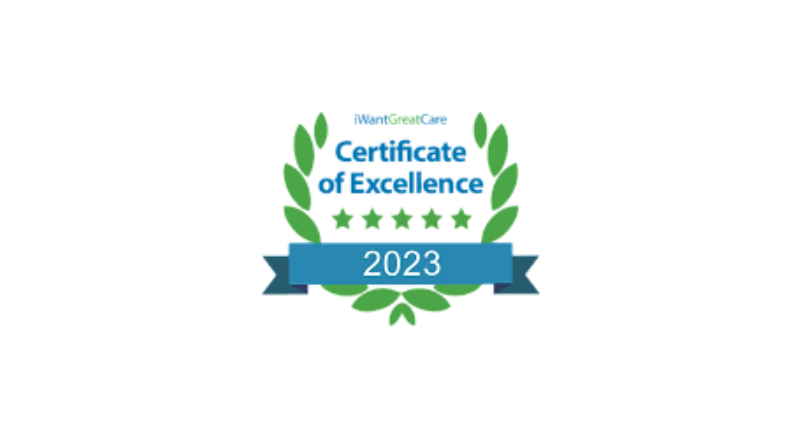
댓글 달기