Personalized Depression Treatment
Traditional treatment and medications do not work for many patients suffering from depression. A customized treatment may be the answer.
Cue is an intervention platform for digital devices that translates passively acquired normal sensor data from smartphones into customized micro-interventions to improve mental health. We analyzed the best-fitting personalized ML models for each individual using Shapley values to discover their features and predictors. This revealed distinct features that changed mood in a predictable manner over time.
Predictors of Mood
Depression is among the most prevalent causes of mental illness.1 Yet, only half of those who have the condition receive treatment1. To improve the outcomes, doctors must be able to recognize and treat patients who have the highest probability of responding to particular treatments.
The ability to tailor depression treatments is one method to achieve this. Researchers at the University of Illinois Chicago are developing new methods for predicting which patients will gain the most from specific treatments. They make use of sensors for mobile phones, a voice assistant with artificial intelligence and other digital tools. Two grants were awarded that total over $10 million, they will use these tools to identify the biological and behavioral factors that determine response to antidepressant medications and psychotherapy.
To date, the majority of research on predictors for depression treatment effectiveness has been focused on sociodemographic and clinical characteristics. These include demographics like age, gender, and education, as well as clinical characteristics such as symptom severity and comorbidities as well as biological markers.
While many of these aspects can be predicted from information in medical records, very few studies have utilized longitudinal data to study predictors of mood in individuals. Many studies do not take into consideration the fact that mood can vary significantly between individuals. Therefore, it is critical to create methods that allow the recognition of individual differences in mood predictors and the effects of treatment.
The team's new approach uses daily, in-person evaluations of mood and lifestyle variables using a smartphone app called AWARE, a cognitive evaluation with the BiAffect app and electroencephalography -- an imaging technique that monitors brain activity. The team will then create algorithms to detect patterns of behaviour and emotions that are unique to each individual.
In addition to these modalities, the team also developed a machine-learning algorithm to model the dynamic factors that determine a person's depressed mood. The algorithm blends the individual characteristics to create an individual "digital genotype" for each participant.
This digital phenotype has been linked to CAT DI scores which is a psychometrically validated symptom severity scale. The correlation was low, however (Pearson r = 0,08, P-value adjusted by BH 3.55 x 10 03) and varied widely between individuals.
Predictors of symptoms
Depression is among the leading causes of disability1 but is often untreated and not diagnosed. In addition, a lack of effective interventions and stigmatization associated with depressive disorders stop many people from seeking help.
To help with personalized treatment, it is crucial to identify predictors of symptoms. Current prediction methods rely heavily on clinical interviews, which aren't reliable and only reveal a few features associated with post pregnancy depression treatment.
Machine learning can improve the accuracy of diagnosis and treatment for depression by combining continuous, digital behavioral phenotypes gathered from smartphones with a validated mental health tracker online (the Computerized Adaptive Testing Depression Inventory CAT-DI). Digital phenotypes are able to are able to capture a variety of distinct actions and behaviors that are difficult to record through interviews and permit continuous, high-resolution measurements.
The study comprised University of California Los Angeles students who had mild to severe Depression Treatment Medicine symptoms who were participating in the Screening and treatment resistant anxiety and depression for Anxiety and Depression program29, which was developed as part of the UCLA Depression Grand Challenge. Participants were directed to online support or to clinical treatment depending on the degree of their depression. Patients who scored high on the CAT-DI scale of 35 or 65 were assigned online support with an online peer coach, whereas those who scored 75 were sent to clinics in-person for psychotherapy.
Participants were asked a set of questions at the beginning of the study concerning their psychosocial and demographic characteristics as well as their socioeconomic status. The questions covered education, age, sex and gender and financial status, marital status as well as whether they divorced or not, current suicidal ideas, intent or attempts, and how often they drank. Participants also rated their degree of post pregnancy depression treatment symptom severity on a scale of 0-100 using the CAT-DI. The CAT DI assessment was conducted every two weeks for those who received online support and weekly for those who received in-person care.
Predictors of Treatment Response
The development of a personalized depression treatment is currently a major research area and many studies aim at identifying predictors that help clinicians determine the most effective drugs for each patient. Pharmacogenetics in particular uncovers genetic variations that affect how the human body metabolizes drugs. This allows doctors select medications that will likely work best for every patient, minimizing the time and effort needed for trial-and error treatments and avoiding any side consequences.
Another approach that is promising is to create prediction models combining information from clinical studies and neural imaging data. These models can be used to identify which variables are most likely to predict a specific outcome, like whether a medication can improve mood or symptoms. These models can be used to predict the response of a patient to a treatment, allowing doctors maximize the effectiveness.
A new generation uses machine learning methods such as the supervised and classification algorithms such as regularized logistic regression, and tree-based techniques to combine the effects of multiple variables to improve the accuracy of predictive. These models have been demonstrated to be effective in predicting treatment outcomes like the response to antidepressants. These models are getting more popular in psychiatry and it is likely that they will become the norm for the future of clinical practice.
The study of depression's underlying mechanisms continues, as do ML-based predictive models. Recent research suggests that depression can be treated is linked to the dysfunctions of specific neural networks. This theory suggests that an individualized treatment for depression will be based upon targeted therapies that restore normal functioning to these circuits.
Internet-based interventions are a way to accomplish this. They can provide an individualized and tailored experience for patients. One study found that a web-based program improved symptoms and improved quality life for MDD patients. Additionally, a randomized controlled study of a customized treatment for depression demonstrated steady improvement and decreased side effects in a significant number of participants.
Predictors of side effects
A major issue in personalizing depression treatment involves identifying and predicting which antidepressant medications will have very little or no side effects. Many patients are prescribed a variety drugs before they find a drug that is both effective and well-tolerated. Pharmacogenetics provides an exciting new avenue for a more efficient and specific approach to selecting antidepressant treatments.
Several predictors may be used to determine the best antidepressant to prescribe, including gene variants, patient phenotypes (e.g. sexual orientation, gender or ethnicity) and co-morbidities. However, identifying the most reliable and valid predictors for a particular treatment is likely to require randomized controlled trials of considerably larger samples than those typically enrolled in clinical trials. This is due to the fact that the identification of interactions or moderators could be more difficult in trials that take into account a single episode of treatment per patient instead of multiple episodes of treatment over time.
In addition, predicting a patient's response will likely require information on the comorbidities, symptoms profiles and the patient's own perception of the effectiveness and tolerability. Currently, only a few easily measurable sociodemographic variables as well as clinical variables are reliably related to response to MDD. These include gender, age, race/ethnicity, BMI, SES and the presence of alexithymia.
The application of pharmacogenetics in treatment for depression is in its beginning stages, and many challenges remain. It is crucial to have a clear understanding and definition of the genetic factors that cause depression, as well as an understanding of a reliable predictor of treatment response. Ethics, such as privacy, and the responsible use of genetic information must also be considered. In the long term the use of pharmacogenetics could offer a chance to lessen the stigma associated with mental health care and improve the treatment outcomes for patients with depression. However, as with all approaches to psychiatry, careful consideration and planning is required. The best method is to offer patients a variety of effective medications for depression and encourage them to speak with their physicians about their experiences and concerns.
Traditional treatment and medications do not work for many patients suffering from depression. A customized treatment may be the answer.
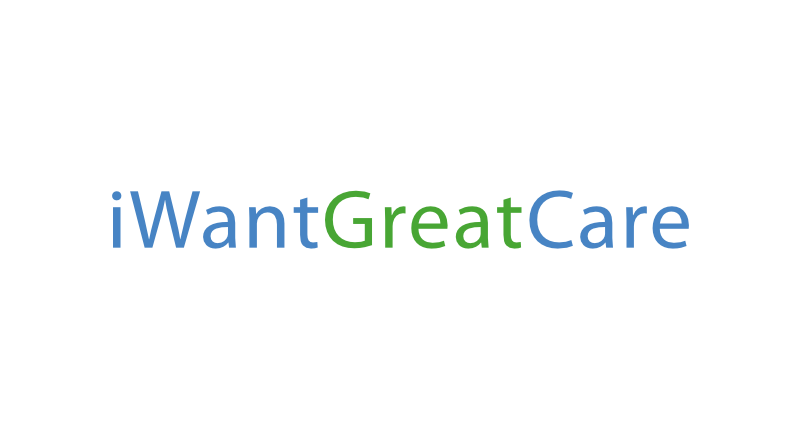
Predictors of Mood
Depression is among the most prevalent causes of mental illness.1 Yet, only half of those who have the condition receive treatment1. To improve the outcomes, doctors must be able to recognize and treat patients who have the highest probability of responding to particular treatments.
The ability to tailor depression treatments is one method to achieve this. Researchers at the University of Illinois Chicago are developing new methods for predicting which patients will gain the most from specific treatments. They make use of sensors for mobile phones, a voice assistant with artificial intelligence and other digital tools. Two grants were awarded that total over $10 million, they will use these tools to identify the biological and behavioral factors that determine response to antidepressant medications and psychotherapy.
To date, the majority of research on predictors for depression treatment effectiveness has been focused on sociodemographic and clinical characteristics. These include demographics like age, gender, and education, as well as clinical characteristics such as symptom severity and comorbidities as well as biological markers.
While many of these aspects can be predicted from information in medical records, very few studies have utilized longitudinal data to study predictors of mood in individuals. Many studies do not take into consideration the fact that mood can vary significantly between individuals. Therefore, it is critical to create methods that allow the recognition of individual differences in mood predictors and the effects of treatment.
The team's new approach uses daily, in-person evaluations of mood and lifestyle variables using a smartphone app called AWARE, a cognitive evaluation with the BiAffect app and electroencephalography -- an imaging technique that monitors brain activity. The team will then create algorithms to detect patterns of behaviour and emotions that are unique to each individual.
In addition to these modalities, the team also developed a machine-learning algorithm to model the dynamic factors that determine a person's depressed mood. The algorithm blends the individual characteristics to create an individual "digital genotype" for each participant.
This digital phenotype has been linked to CAT DI scores which is a psychometrically validated symptom severity scale. The correlation was low, however (Pearson r = 0,08, P-value adjusted by BH 3.55 x 10 03) and varied widely between individuals.
Predictors of symptoms
Depression is among the leading causes of disability1 but is often untreated and not diagnosed. In addition, a lack of effective interventions and stigmatization associated with depressive disorders stop many people from seeking help.
To help with personalized treatment, it is crucial to identify predictors of symptoms. Current prediction methods rely heavily on clinical interviews, which aren't reliable and only reveal a few features associated with post pregnancy depression treatment.
Machine learning can improve the accuracy of diagnosis and treatment for depression by combining continuous, digital behavioral phenotypes gathered from smartphones with a validated mental health tracker online (the Computerized Adaptive Testing Depression Inventory CAT-DI). Digital phenotypes are able to are able to capture a variety of distinct actions and behaviors that are difficult to record through interviews and permit continuous, high-resolution measurements.
The study comprised University of California Los Angeles students who had mild to severe Depression Treatment Medicine symptoms who were participating in the Screening and treatment resistant anxiety and depression for Anxiety and Depression program29, which was developed as part of the UCLA Depression Grand Challenge. Participants were directed to online support or to clinical treatment depending on the degree of their depression. Patients who scored high on the CAT-DI scale of 35 or 65 were assigned online support with an online peer coach, whereas those who scored 75 were sent to clinics in-person for psychotherapy.
Participants were asked a set of questions at the beginning of the study concerning their psychosocial and demographic characteristics as well as their socioeconomic status. The questions covered education, age, sex and gender and financial status, marital status as well as whether they divorced or not, current suicidal ideas, intent or attempts, and how often they drank. Participants also rated their degree of post pregnancy depression treatment symptom severity on a scale of 0-100 using the CAT-DI. The CAT DI assessment was conducted every two weeks for those who received online support and weekly for those who received in-person care.
Predictors of Treatment Response
The development of a personalized depression treatment is currently a major research area and many studies aim at identifying predictors that help clinicians determine the most effective drugs for each patient. Pharmacogenetics in particular uncovers genetic variations that affect how the human body metabolizes drugs. This allows doctors select medications that will likely work best for every patient, minimizing the time and effort needed for trial-and error treatments and avoiding any side consequences.
Another approach that is promising is to create prediction models combining information from clinical studies and neural imaging data. These models can be used to identify which variables are most likely to predict a specific outcome, like whether a medication can improve mood or symptoms. These models can be used to predict the response of a patient to a treatment, allowing doctors maximize the effectiveness.
A new generation uses machine learning methods such as the supervised and classification algorithms such as regularized logistic regression, and tree-based techniques to combine the effects of multiple variables to improve the accuracy of predictive. These models have been demonstrated to be effective in predicting treatment outcomes like the response to antidepressants. These models are getting more popular in psychiatry and it is likely that they will become the norm for the future of clinical practice.
The study of depression's underlying mechanisms continues, as do ML-based predictive models. Recent research suggests that depression can be treated is linked to the dysfunctions of specific neural networks. This theory suggests that an individualized treatment for depression will be based upon targeted therapies that restore normal functioning to these circuits.
Internet-based interventions are a way to accomplish this. They can provide an individualized and tailored experience for patients. One study found that a web-based program improved symptoms and improved quality life for MDD patients. Additionally, a randomized controlled study of a customized treatment for depression demonstrated steady improvement and decreased side effects in a significant number of participants.
Predictors of side effects
A major issue in personalizing depression treatment involves identifying and predicting which antidepressant medications will have very little or no side effects. Many patients are prescribed a variety drugs before they find a drug that is both effective and well-tolerated. Pharmacogenetics provides an exciting new avenue for a more efficient and specific approach to selecting antidepressant treatments.
Several predictors may be used to determine the best antidepressant to prescribe, including gene variants, patient phenotypes (e.g. sexual orientation, gender or ethnicity) and co-morbidities. However, identifying the most reliable and valid predictors for a particular treatment is likely to require randomized controlled trials of considerably larger samples than those typically enrolled in clinical trials. This is due to the fact that the identification of interactions or moderators could be more difficult in trials that take into account a single episode of treatment per patient instead of multiple episodes of treatment over time.
In addition, predicting a patient's response will likely require information on the comorbidities, symptoms profiles and the patient's own perception of the effectiveness and tolerability. Currently, only a few easily measurable sociodemographic variables as well as clinical variables are reliably related to response to MDD. These include gender, age, race/ethnicity, BMI, SES and the presence of alexithymia.
The application of pharmacogenetics in treatment for depression is in its beginning stages, and many challenges remain. It is crucial to have a clear understanding and definition of the genetic factors that cause depression, as well as an understanding of a reliable predictor of treatment response. Ethics, such as privacy, and the responsible use of genetic information must also be considered. In the long term the use of pharmacogenetics could offer a chance to lessen the stigma associated with mental health care and improve the treatment outcomes for patients with depression. However, as with all approaches to psychiatry, careful consideration and planning is required. The best method is to offer patients a variety of effective medications for depression and encourage them to speak with their physicians about their experiences and concerns.

댓글 달기